Census is excited to present our first dbt package dbt_census_utils, featuring six macros that bypass the most tedious aspects of readying your data for activation. In the past, we’ve published articles on common data modeling requirements, like extracting email domains from email addresses, but why not just…do the transformation for you? 🪄
You can peek at the package’s source code if you want to know the nitty-gritty details on how it’s done, but the TL;DR is: Our new dbt_census_utils package helps you make your data ready to activate in over 200 SaaS tools.
The package features common macros for Reverse ETL use cases, such as identifying personal emails vs. business emails, cleaning names for advertising destinations, and standardizing country codes.
The best part? Even if you don’t use Census today, you can use these macros to save time and teach you new tricks in dbt. 🙌
Before we dive in, we (the Census Team) have to give a huge shout-out to Stephen Ebrey, founder of Sawtelle Analytics and OA Club Data Champion, for his work building this package with us.
dbt packages make your life easier
A data scientist joining a new company can quickly do advanced analysis using Python libraries like pandas, numpy, and matplotlib, but until recently, data engineers and analysts generally wrote a ton of custom SQL. This caused data teams to expand in size to service all the use cases that a modern data stack can provide, like business intelligence, product analytics, marketing attribution, and data activation.
dbt packages make it easier to build, manage, and test data transformations using open-source code. This brings analytics engineering in SQL up to par with data science and software engineering by letting you skip solving the same old problems as everyone else. Get to the juicy particulars of your business – faster!
What’s unique about these dbt packages for data activation?
Data activation has some specific challenges that traditional BI doesn’t have, like needing to match the customer parameter guidelines of a destination. Misformatting names or emails can cause records to be rejected by the destination’s API, and formatting them too much can interfere with user-matching processes. For instance, if you unnecessarily change João to joao, Google Ads may not realize the user was already shown an ad.
Also, data activation can confuse operational teams if we’re not clearly delineating internal vs. external users. For example, internal employees must be differentiated from those using a work email as well as users coming from the same email domain. You don’t want a salesperson to get excited about a PQL in Salesforce when it’s actually just a developer working for your company. We’ve solved these problems before, and we want to pass on our solutions to you!
Useful macros for just about anyone
The extract_email_domain, is_personal_email, is_internal, and get_country_code macros are important fields to use when sending data to third-party systems and traditional BI. For example, if you have a QA tester who made 30 purchases, you wouldn’t want to rate them as a highly qualified lead in Salesforce, or pay to remarket to them on Instagram. But you wouldn’t want to count them in your average LTV score in Tableau or Looker.
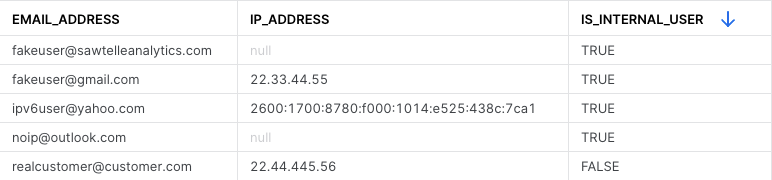
You might not want to prioritize Zendesk requests from fred@gmail.com as highly as ones from ceo@hotnewstartup.com, and you also might not want to count gmail addresses when calculating conversion rate in Mode. Plus, it’s helpful to link sara@client.com with amit@client.com, both in HubSpot and your count of unique customers on the executive dashboard!
Another common use case is that you may have some sources that report countries in names and some in codes, and you want to standardize them all with get_country_code before building reports or sending them off to destinations.
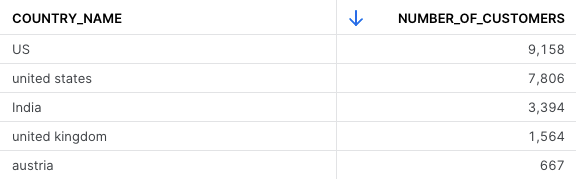
With get_country_code, the entries above become:
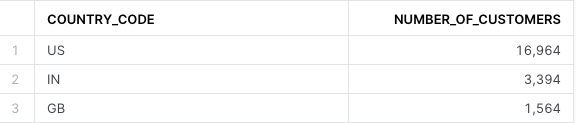
Use macros to standardize user data specifically for services like Google and Facebook Ads
The clean macro lowercases, removes spaces and symbols, and removes special characters from names, so they won’t get rejected from destinations like Facebook audiences. This is very useful with Census or your own reverse ETL pipelines to these destinations, but traditional BI does not require São Paulo to become saopaulo.

Useful macros for GA4 customers
The parse_ga4_client_id was a request from a Census customer who had trouble standardizing their GA 4 client IDs. It lets you separate the unique ID part of the client ID or the timestamp part (which represents the user’s first visit). All GA4 customers can benefit from this cleaning logic, so we’ve added it to the package as well!
A look behind the scenes
If you’re curious about dbt packages, here’s a brief overview of how we created this package:
Info gathering
Stephen combed through dozens of dbt packages on the package hub to see what problems were already solved, who made the best packages, and how much was company sponsored vs. community-contributed. He looked at how dbt packages implemented tests with methods like dbt_utils.equality and how they supported multiple data warehouses with dispatch and cross-database macros.
Some smaller packages are only tested on one or two warehouses. We decided to support Redshift, Snowflake, and BigQuery, which meant setting up multiple test warehouses, dbt profiles, and learning how to link and unlink different dbt adapters.
Feature specifications
Stephen first got the idea of creating a dbt package for Census specifically when he got some advice on how to get city names to be accepted by Facebook, with a very-ugly line of SQL that looks like the following:
regexp_replace(translate(lower(name),'ůțąðěřšžųłşșýźľňèéëêēėęàáâäæãåāîïíīįìôöòóœøōõûüùúūñńçćč','utaoerszutssyzlneeeeeeaaaaaaaaiiiiiioooooooouuuuunnccc'),'[^a-z]','')
With that, he thought, “Surely, a clean() macro would be so much more elegant!” Then he started seeing other uses for macros from Census articles and working with clients. Stephen also made sure they weren’t already provided in dbt_utils or any other packages.
Implementation
Writing a dbt macro is simple. Making sure it works for multiple warehouses, on the other hand, is tricky! Redshift, BigQuery, and Snowflake handle arrays very differently (*cough* Redshift does it the worst *cough*). And with the is_internal macro, we needed to use variables so customers could specify their domain and any tables they had with lists of internal users.
With the extract_email_domain, is_personal_email, is_internal, and get_country_code macros, we had to compile lists of standardized country name formats and common personal email domains. Finally, Stephen ensured each macro had an integration test so it worked on all platforms and that changes did not cause them to break.
Documentation
A dbt package needs a readme, dbt docs, an entry on the dbt package hub, and issue and PR templates! As a final step, Stephen did the holy work of writing a ton of documentation. We’ll release more packages and a lot of this work in dbt_census_utils can be reused, but if you’re trying to write a package yourself, leave time for this step.
We want your feedback
dbt_census_utils is our first dbt package, and you can request other macros that help wrangle data for use on Census with a Github Issue, or try to implement it yourself in a pull request. We also monitor for questions and comments in the #tools-census channel in dbt Slack, as well as on the Operational Analytics Club Slack.
📑Check out the package here, then put dbt_census_utils to the test for yourself with Census. Book a demo to get started.
If you’d like to learn more about the work that went into building this package, and some of the challenges Stephen worked through in the process, join us for his webinar June 5 at 10 am PT.
👉 Want to chat about this package? Got other burning data questions? Head over to the Operational Analytics Club to join tons of other data-savvy folks in their quest for more knowledge.