Traditional data culture wields data teams to compile metrics and dashboards that leaders use to drive business decisions. Even with all that data at their disposal, however, there’s often disagreement among data leaders and stakeholders regarding which data should drive which initiatives. This typical roadblock lends itself to the question: should this traditional methodology be the other way around to properly leverage data for real business success? 🤔
Erik Bernhardsson, former CTO of Better and the brain that built Spotify’s music recommendations feature, thinks so. In his opinion, the data team should be a “critical thinking” unit, used to investigate and explore which metrics would make the biggest impact on the company’s goals. Because, as Erik points out, when data folks are treated as curators who simply pull out the metrics they’re asked for, they’re not being used to their full potential.
“I’d rather smash that iteration loop and give decision-making ability to people deeper in the data,” Erik said. “Then, rather than wasting time building dashboards, you could have decisions made at a local level that incorporates a lot more information.”
As a data leader himself, Erik’s contention is not that executives outside the data team make bad decisions. Instead, it’s that properly leveraged data can not only answer business questions but can actually clarify which questions to ask.
For example, leadership might be interested in reducing user acquisition costs, but they don’t know where to start. How they should track the costs? Should they track the cost per lead, cost per transaction, or some other measure?
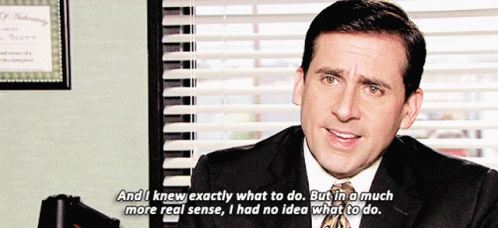
Call us crazy, but by loosening the reins and allowing data analysts to model the question before trying to answer it, leadership can get an identified, substantiated metric to optimize for.
“I wonder if the top-level metrics business units care about are actually less important than the day-to-day things product managers care about,” Erik mused. “Because that’s what produces actionable insights.”
Tracking the right metrics
To build a truly data-driven company, leaders need to identify quality metrics that can measurably impact their business. According to Census founder and CEO Boris Jabes, workers who aren’t given a goal will come up with their own – and it might not align with leaders’ vision for the company.
“When people walk in every morning, they need to ask: ‘How can I make the company better today?’” Boris said. “If they don’t know how the company defines that, they define it in terms of their job and their tools, and they pursue less important, intermediate outcomes rather than the ultimate outcome.”
In the early days of Spotify, the goal was simply to grow daily active users. Every team throughout the company was aware of this goal and knew that was the outcome they were working toward. Leaders had faith that if the company could add users every day, everything else would fall into place.
“What you care about in the long term is shareholder value, but you can’t see that. You can’t measure it,” Erik said. “You have to pick metrics as proxies – things you can A/B test, that are hard to game, and that don’t have short-term fluctuations out of your control.”
Once leaders have shared the ultimate goal, it’s time for the hard part: get out of the way and let teams develop innovative ways to reach it. Letting teams set their own intermediate metrics with enough room for experimentation is a crucial part of managing creative people.
When Erik started at Spotify, the small, original data team was tasked with organizing and cleaning up the data framework. At that time, developing artificial intelligence and machine learning that could recommend new music wasn’t even a blip on the company’s radar.
Erik, however, encouraged the people on his team to research and experiment with any idea they were convinced would further the goal of adding users. He also recognized that earning upper management buy-in was a two-fold process, so he regularly reported results that added value to the business, proving the value of innovation while emphasizing that the day-to-day needs of the business weren’t being neglected along the way.
“You need really good KPIs to show you’re delivering outcomes,” Boris agreed. “You have to work on the basic problems to prove you can do the work. Before you earn the trust to work on your dream project, you first have to do the things that have the highest value for the business.”
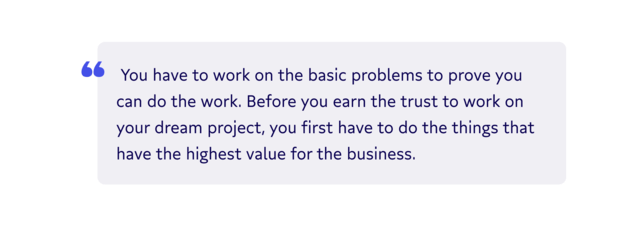
For this purpose, the right reporting structure is key. You need to make sure data teams have the bandwidth to drive toward company success rather than just putting out fires for other departments. Data teams are a uniquely-shared resource in a way that few other departments are, so they must be distinctly protected from collaboration burnout.
Though neither works especially well, there are two standard reporting structures for data teams: centralized and decentralized models. In the centralized model, everyone in the company funnels tickets to a single data team, leading to strained resource allocation and departmental squabbling over whose project should get priority.
In the decentralized model, each business unit has its own version of a data team which, in turn, creates personnel problems. Leaders without a data background don’t know who to hire or how to measure performance, while ambitious data professionals don’t want to work in an environment where it will be difficult to grow and challenge their skills.
So, how do you get effective data and avoid this sticky situation? Cue the embedded model, where whole successful data teams report to a centralized head of data, but the individual team members perform day-to-day tasks in their assigned business units. Much like a newspaper might assign one reporter to a city hall beat and one to a performing arts beat, data professionals do day-to-day tasks within product or marketing teams without actually joining those units’ teams.
Building an innovative team
Once people have proven they can do the work, rewarding them with the freedom to innovate can produce the most value. At first, Erik observed, Spotify was growing just fine as a music player. When music became commoditized, though, the recommendation algorithm his team developed became the differentiator that put Spotify on a different level than its competitors.
“The most successful data people aren’t the ones interested in tools – they’re the ones with a deep curiosity about the business,” Erik explained. “To build a successful startup you need people who care about what the business is trying to do.”
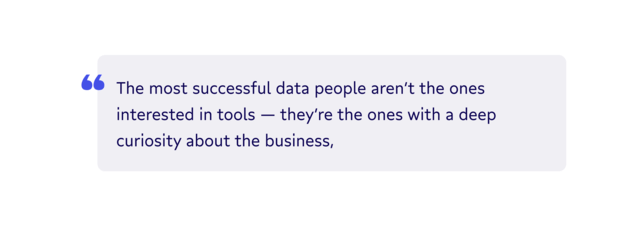
Early in his career, Erik considered himself a tool-oriented engineer. He had a passion for algorithms and believed that building them would bring him the most career satisfaction – until he found himself working at a startup. There, he realized that algorithms were simply means to an end, and what he really cared about was building a successful business.
Erik cautions startups and early-stage businesses against building a data team of people with too much love for their trade tools. A data professional whose career ambition is to deepen their knowledge of functional programming or machine learning is fundamentally misaligned with what an early-stage business needs: creative people who are more interested in the outcome than the process.
The application of data in business is developing so rapidly that businesses are struggling to adapt organizational charts fast enough to accommodate it. The success of innovators like Spotify proves the data world has more to offer than advising leaders on Option A or Option B.
Businesses have used the structure of established teams like engineering as a template to build their data operations – but the field is fast outgrowing these borrowed templates. High-performing companies will diverge from the norm, adapting their structure to give data teams more room to innovate and influence their evolution.
Want to learn more? Listen to the full conversation between Boris and Erik here. Got thoughts and opinions about this topic? Join the conversation around this, and many other data best practices, in ✨ The Operational Analytics Club. ✨