Building a modern data stack doesn’t require a lot of up-front effort. In fact, one of the biggest mistakes you can make when transitioning to a modern data stack is trying to do it all at once as a Goliath task.There are a lot of our favorite folks in the data space that agree with this take (in fact, a bunch of them recently talked about building the modern data stack in a recent Mixpanel’s recent webinar). By starting small, you lean into one of the modern data stack’s main strengths: its modularity. In the process, you’ll steadily construct a data infrastructure that’s efficient and customized to your business’s exact needs, all without stressful workflow disruptions and difficult buy-in conversations. So, start small. The journey to a modern data stack (and a leap into better data) starts with one small optimization.
It’s the modularity of the modern data stack that makes it exciting
The modern data stack is unique because it’s much more modular than stacks of the past. By “modular,” I mean that each tool serves a distinct purpose, mutually exclusive to the other tools that support it. This modularity is where a lot of the modern data stack’s strength comes from and is why it may seem feasible to shift your entire data stack all at once.
We think of the modern data stack as a body, with a reverse ETL tool (like Census 👋) serving as the spine of the nervous system. Individually, each type of tool has a specific job that none of the others can do, while collectively, they all function as one unit.
Domo shows a good example of non-modular design: it’s a product that dabbles in visualization, data transformation, data ingestion, and more. With non-modular tools like Domo, you typically use only a handful of its features, which means you’re paying for more than you use. If you do use all of its features, there are bound to be areas where functionality could be improved with a more niched-down product. All this means your data team has to create time-intensive workarounds or make do with sub-par functionality.
Gather a bunch of these non-modular tools into one stack, and you get a lot of overlap, redundancy, and waste. What’s worse, it’s hard to rethink which tools you use without rethinking the whole stack because they’re all tangled together like a headphone cord left in your pocket.
Modularity, on the other hand, leads to simplicity. Because each tool focuses solely on its own area of expertise, you know exactly what each tool in the stack is doing and why. This typically leads to tools with better ROI (i.e., they’re efficient at accomplishing what you pay for) that are easier to implement because of their specialized scope. Plus, if you need to swap out a tool, you’re not rethinking the entire stack; you’re just changing one part.
Drizly’s presentation at Fivetran’s 2020 Modern Data Stack Conference is a great encapsulation of why you should move slowly and the impact a modern data stack can have on an organization. Drizly started their transition to a modern data stack in late 2019 by focusing on the processes and teams data could support best, and by mid-2020, they were most of the way through their data journey. As an alcohol delivery service, their business boomed during the COVID-19 pandemic, and with this new data stack, they were not only able to adapt but also simultaneously build a data foundation for their organization. All of this started with a single step toward the modern data stack.
The prospect of having a set of modular, best-in-class tools in your data stack is exciting for a lot of businesses we talk to (and not just because it puts them in the same class as companies like Drizly). Many of them want to start right away and transition to new tools and systems all at once.
But I encourage you to press pause, start small, and really think through the transition process to a modern data stack.
Just because you can move fast doesn’t mean you should
Compared to data infrastructure from even five years ago, a modular modern data infrastructure is very quick and simple to set up. However, you should still take your time in creating a modern data infrastructure so you can build it to your specifications.
Seth Rosen at Hashpath proved in late 2020 that someone with minimal data experience could set up a full analytics stack in around 45 minutes. In the past, something similar would’ve likely taken at least a two-week sprint. While Seth’s example is more a proof of concept than a full playbook, his point remains: “The infrastructure of a modern analytics stack is now the easiest part.”
The hard part of building the modern data stack is making sure your new tooling fits your specific use case.
To take a page out of Drizly’s data book again: Move slowly and carefully evaluate each tool you add to your stack. It took Drizly about 18 months to make the transition from their old data stack powered by Redshift and Domo to their new, modern data stack powered by Snowflake, dbt, and Census that fits their exact business needs.
Drizly started small by focusing on standardizing and simplifying their modeling process. The Drizly data team could see what impact an improved modeling process had before moving on to identify their next problem: data warehouse scalability (they switched from Redshift to Snowflake). On this foundation of a standardized modeling process, Drizly could then methodically pick and choose what to prioritize next.
By measuring the success of each pilot process before moving into the next step, the Drizly team knew that they were building on foundation that would support each subsequent goal.
Focus on simplifying specific processes first
Most data teams we talk to have one (or a dozen) processes that drive them up the wall—from version control to dealing with a flood of ad-hoc requests. Rather than casting a wide net to solve all these pain points at once, start implementing your modern data stack where your team experiences the most acute discomfort.
This means picking one process to optimize that solves a specific pain point, then finding the right tool for that optimized process and implementing it first. In Drizly’s case, their data team modeled data within Domo, which was a huge headache.
Emily Hawkins, data infrastructure lead at Drizly, explained, “There was no real version control. There were datasets in Domo that were labeled ‘_donotuse’ and ‘_WIP.’ It was not a great process to figure out what had changed or what was accurate. That was one of the first things we wanted to tackle.”
So, the first phase of their transition to a modern data stack focused solely on moving modeling out of Domo and into dbt. This first phase took about five months to complete and allowed Drizly’s data team to simplify and standardize their modeling. If you need some inspiration, here are three ways to solve the immediate aches and pains of most teams:
- Get CS reps the data they need faster. Great customer experience helps reduce churn and has a direct impact on growth and revenue. Use a reverse ETL tool, like Census, to pull data out of a data warehouse and into Zendesk. Start with just one set of data, like how many users are active in one account.
- Heatmap data destinations. Look into where your data team sends data most often to determine what processes you should prioritize first. For instance, if it’s Salesforce, talk to the sales team and standardize that data flow.
- Track how your data team uses data. Knowing the numbers behind how data teams use data will help you find process bottlenecks that need simplification or standardization. For example, Drizly tracked event data volume per day, commits per day, and deploys per week.
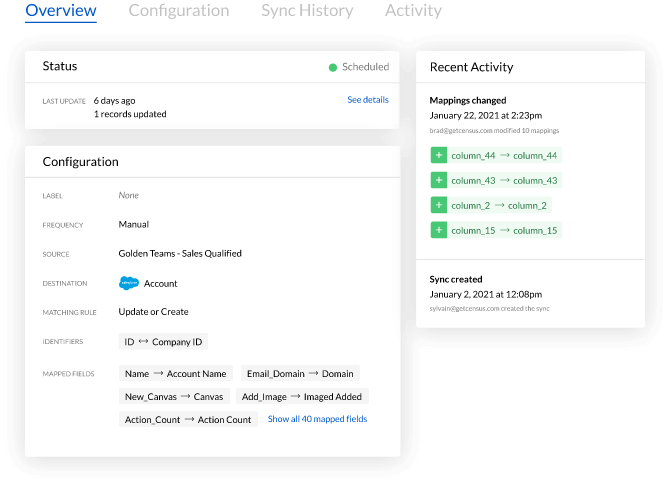
Moving modeling out of Domo and into dbt, while a good example of simplification, is probably still too big of a process to start with for many data teams. Don’t be afraid to go even smaller. The goal should be to hone in on one specific process and simplify it with a specialized tool.
Quick wins build buy-in for your dream data stack
Starting small with the core processes and workflows within your organization allows you to build a series of quick wins. These quick wins help prove to executives that your vision for how your organization uses data has merit.
A reverse ETL tool like Census puts data into the hands of your stakeholders so they can make daily, data-informed decisions that impact the bottom line. To dive deeper into what it means to build a modern data stack, check out our startup guide to the modern data stack.