If you’re part of any online data communities or a subscriber to the many data newsletters out there in the world, you’ve probably seen a few articles trying to boil down the “right” answer to “What is data as a product?”.
Before I get into all the nit-picking and hot takes, I think we can all agree on one thing: Like many debates among data practitioners about best practices and methodologies, there’s no one clear, definitive “right answer.” In the end, what matters isn’t necessarily the exact dictionary definition of data as a product, but rather that data teams work diligently to find processes and systems that help them advocate for the importance of data on a wider, organizational level.
If your organization has already defined and put data as a product principles into practice, kudos to you! However, if your organization has yet to put data as a product principles into practice, this article may help you standardize DataOps best practices, as well as secure the data team the seat they deserve at the organizational table (or, at the very least, get the conversation going).
In this article, I’ll break down some of the top content around data as a product, and then share my opinion of its definition. First, let’s summarize some of the top content surrounding data as a product.
The definition of “data as a product”: A summary so far
A few weeks back, our community members had a hearty debate about what data as a product meant and how important it really was. I decided to dive deep into the current opinion/writing on the topic and pulled together the main hot takes below so you can save yourself a few hours of reading. Generally speaking, the definitions fall into the following four categories:
- Data as a product is about … key product development principles.
- Data as a product is about … providing data to stakeholders automatically.
- Data as a product is about … applying the principles of product thinking.
- Data as a product is about … all the tools, processes, and people that go into it.
Data as a product is about … key product development principles.
Under this definition, data as a product is explained as the concept of applying key product development principles (such as identifying and addressing unmet needs, agility, iterability, and reusability) to data projects. This belief has popped up in the writings of Jedd Davis, Dave Nussbaum, Kevin Troyanos: Approach your data with a product mindset (HBR).It notably hinges on two milestone outcomes from the data team:
- The data team can understand and apply the product development principles above.
- The data team has developed point solutions that solve the specific needs of their stakeholders.
In milestone two, a point solution is similar to data as a service (DaaS). It uses datasets and techniques to solve a specific problem in an organization, but they don’t satisfy the final principle of product development: reusability.
Once a data team has abstracted the underlying principles of successful point solutions and reused them to solve an array of business problems, they have achieved data as a product.
Data as a product is about … providing data to stakeholders automatically.
Under this definition, data as a product is about the concept of providing data to stakeholders in an automated fashion to facilitate good decision-making. You’ll know this definition if you subscribe to Justin Gage’s newsletter, in which he wrote about data as a product vs data as a service.
Unlike other authors, Justin has a contrarian view of data as a product. He believes that data professionals who specialize in DaaS are rarely capable of achieving DaaP, and they shouldn’t be expected to achieve both. Instead, Justin argues that:
- DaaS professionals have specific domain expertise (marketing, finance, product, etc.) and are focused on providing insights.
- DaaP professionals are engineers. They are focused on building processes and data pipelines and delivering rows/columns of data to facilitate good decision-making.
Additionally, Justin shares some challenges that come with DaaP professionals. Data professionals who are asked to specialize in DaaP are difficult to hire and can get bored because their jobs seem less strategic than DaaS or, as he puts it, “Being a member of a data team that just provides data is boring AF and difficult to hire.”
His chart below shows the time & value tradeoff of DaaP vs DaaS, which illustrates that DaaS provides specifically more value, but it requires more time.
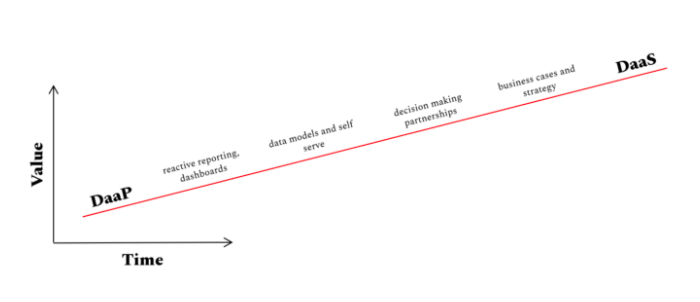
In his final remarks, Justin gives advice to organizations deciding between DaaS and DaaP: “Be intentional about the purpose of the data team, your roadmap for changing that purpose, and who exactly you’re going to hire to get it done.”
Data as a product is about … applying the principles of product thinking.
Data as a product is a mindset that applies the principles of product thinking to create data products. This should sound familiar if you’re a fan of Xavier Rigol and his piece Data as a product vs data products.
This belief is quite similar to the HBR article, but Xavier explains data as a product by first breaking down two essential concepts: product thinking and data products:
- Product thinking: A mindset that’s outcome-oriented, business-capability aligned, long-lived, and cross-functional with the intention to solve problems and improve business outcomes. Additionally, there should be a focus on discoverability, security, explorability, understandability, trustworthiness, etc.
- Data products: Using “raw data, derived data, [and] algorithms” to automate and/or guide decisions to improve business outcomes.
Xavier's article is less of an opinion piece and more of an academic exercise. He doesn’t share why companies should or should not apply the data as a product mindset. Instead, he simply defines data as a product based on his research and findings.
Data as a product is about … all the tools, processes, and people that go into it.
Similar to Xavier’s definition above, this take on DaaP is all about mindset. It defines data as a product as a shared mindset across the entire company that data is, indeed, a product (a bit of a definition within a definition, I know). This belief has popped up in the writings of Emilie Schario & Taylor Murphy: Run your data team like a product team.
Under this view, every piece of data, the tools used to generate, access, and analyze, are integrated together as one big data product. Any internal tool used to make a decision is a feature of the data product.
You can think about it with Legos if you want to get creative here (as Emilie and Taylor did in their original LO piece). Each internal process and tool is, by itself, an individual lego, but united together they build and fortify a larger data product (like a fancy Lego lair).
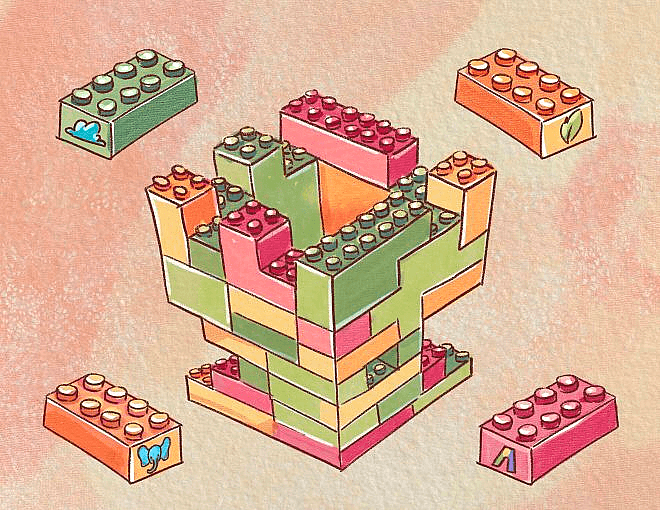
Emilie and Taylor's definition of data as a product appears to be quite different from the HBR, Justin Gage, and Xavier Rigol articles. The key difference is they simply call all company data data as a product, with the individual tools being features of data as a product while other articles are calling the individual features data as a product.
However, the mindset required to achieve data as a product is the still same: Apply key product development principles (Identifying and addressing unmet needs, agility, iterability, and reusability) to data projects.
OK, neat. So what is the real definition of data as a product?!
Each article above has slight differences in the definition and purpose (or lack thereof) of data as a product. After reading through each article and leaning on some of the internal expertise here at Census, I believe that this is the best definition:
Data as a product is the concept of applying key product development principles (Identifying and addressing unmet needs, agility, iterability, and reusability) to data projects.
Fundamentally, data as a product is a concept, or methodology, about how data teams can create value in their organizations. The general belief is that applying product management principles to data teams will make data work more valuable and scalable, qualities that have been lacking in the data community for years.
We believe this is the best definition because it encompasses key product development principles and their application to data projects. Too often do data teams get stuck in the support ticket style trap. Data teams focus on solving here and now problems without thought of applying product management principles to create sustainable solutions. If we apply product management principles and create the tooling and solutions that allow our end-users to serve their stakeholders, our data will be significantly more valuable.
As I mentioned above - like many debates among practitioners about best practices and methodologies, there’s no one clear, definitive “right answer.” In the end, what matters isn’t necessarily the exact dictionary definition of data as a product, but rather that data teams are working to find processes and systems that help them advocate for the importance of data on a wider organizational level.
I'd love to hear your take on this! Feel free to “@” me on Twitter or the Operational Analytics Club with any counter opinions or hot takes.