Churn Prediction Models: Retain Customers
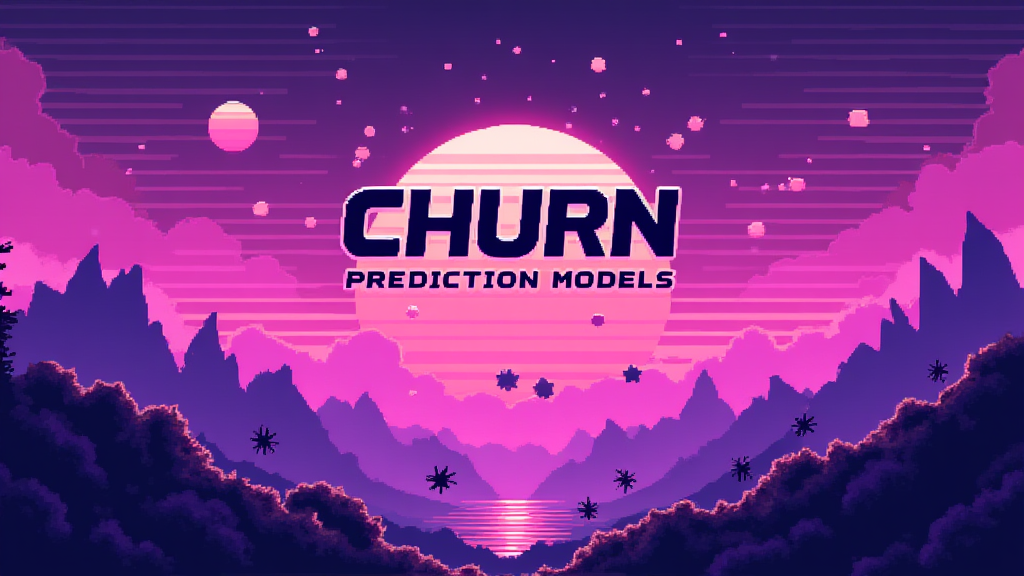
Published on: October 01, 2024
Churn prediction models are sophisticated analytical tools used by businesses to forecast which customers are likely to stop using their products or services. These models leverage historical data, customer behavior patterns, and machine learning algorithms to identify at-risk customers before they actually churn.
Why Churn Prediction Models Matter 🔍
In the competitive landscape of Sales and Marketing Operations, retaining existing customers is often more cost-effective than acquiring new ones. Churn prediction models play a crucial role in:
- Proactively identifying customers at risk of churning
- Enabling targeted retention strategies
- Optimizing resource allocation for customer retention efforts
- Improving overall customer lifetime value
Key Components of Churn Prediction Models
Effective churn prediction models typically incorporate several key elements:
1. Data Sources 📊
- Customer demographics
- Usage patterns
- Purchase history
- Customer support interactions
- Engagement metrics
2. Machine Learning Algorithms 🤖
Common algorithms used in churn prediction models include:
- Logistic Regression
- Random Forest
- Gradient Boosting
- Neural Networks
3. Feature Engineering ⚙️
Creating relevant features from raw data to improve model accuracy.
4. Model Evaluation Metrics 📈
Key metrics for assessing model performance:
- Accuracy
- Precision
- Recall
- F1 Score
- Area Under the ROC Curve (AUC)
Implementing Churn Prediction Models in Practice
To effectively implement churn prediction models in your organization:
- Collect and prepare data: Gather relevant customer data from various sources and ensure its quality.
- Choose appropriate algorithms: Select machine learning algorithms that best fit your data and business needs.
- Train and validate the model: Use historical data to train your model and validate its performance.
- Deploy and monitor: Implement the model in your operations and continuously monitor its performance.
- Act on predictions: Develop targeted retention strategies based on model insights.
Challenges and Considerations 🚧
While churn prediction models offer significant benefits, they also come with challenges:
- Data quality and availability
- Model interpretability
- Balancing precision and recall
- Adapting to changing customer behavior
- Ethical considerations in data usage
The Impact of Churn Prediction Models
Effective implementation of churn prediction models can lead to significant improvements in customer retention and business performance. For example:
Metric | Average Improvement |
---|---|
Customer Retention Rate | 5-15% |
Customer Lifetime Value | 10-25% |
Revenue from Retained Customers | 15-30% |
By leveraging churn prediction models, businesses can not only reduce customer attrition but also enhance overall customer satisfaction and loyalty.
Integrating Churn Prediction Models with Other Tools
To maximize the impact of churn prediction models, integrate them with other tools in your Sales and Marketing Operations stack:
- CRM systems for centralized customer data management
- Customer success platforms for targeted interventions
- Marketing automation tools for personalized retention campaigns
- Business intelligence dashboards for real-time monitoring
As you consider implementing churn prediction models in your organization, ask yourself:
- What data sources do we currently have available for churn prediction?
- How can we integrate churn predictions into our existing customer retention strategies?
- What specific actions can we take based on the model's predictions?
- How will we measure the success of our churn prediction and prevention efforts?
By addressing these questions, you'll be well on your way to leveraging churn prediction models to boost customer retention and drive business growth.