Customer Sentiment Analysis: Decoding Emotions
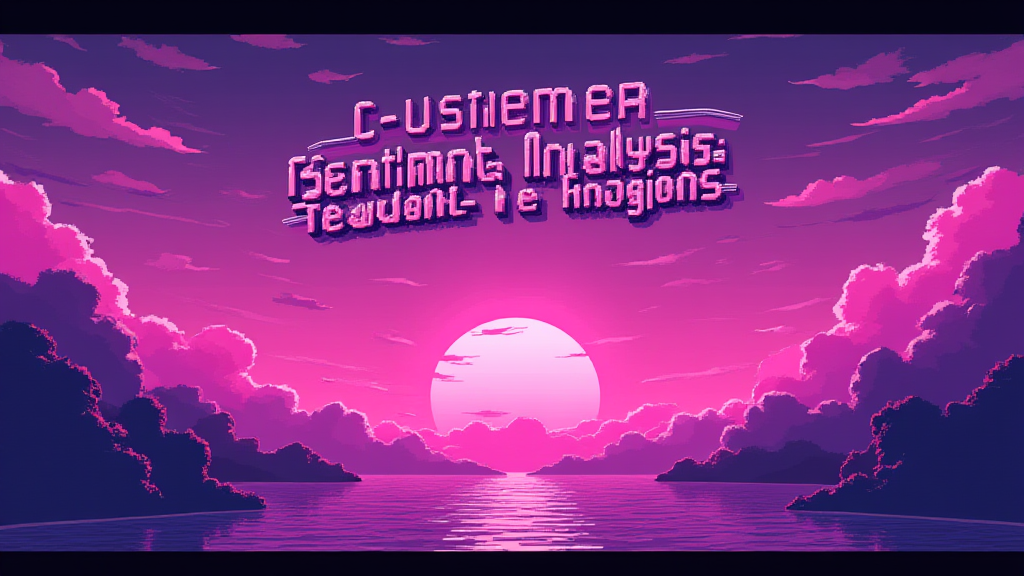
Published on: October 01, 2024
Customer Sentiment Analysis is a powerful technique that uses natural language processing (NLP) and machine learning to systematically identify, extract, and analyze the emotional tone behind customer interactions. This process helps businesses understand how their customers feel about their products, services, or brand, providing invaluable insights for improving customer experience and driving business growth. 📊🔍
Why Customer Sentiment Analysis Matters
In today's competitive business landscape, understanding customer sentiment is crucial for:
- Identifying pain points in the customer journey
- Predicting customer behavior and preferences
- Improving product development and service delivery
- Enhancing customer satisfaction and loyalty
- Mitigating potential crises before they escalate
Key Components of Customer Sentiment Analysis
Effective sentiment analysis involves several key elements:
1. Data Collection 📥
Gathering customer feedback from various sources, including:
- Social media posts
- Customer reviews
- Support tickets
- Surveys and feedback forms
- Call center transcripts
2. Text Processing 🔤
Cleaning and preparing the collected data for analysis, including:
- Removing irrelevant information
- Correcting spelling and grammar
- Tokenization (breaking text into individual words or phrases)
3. Sentiment Classification 📊
Categorizing the processed text into sentiment categories, typically:
- Positive
- Negative
- Neutral
4. Analysis and Visualization 📈
Interpreting the results and presenting them in an easily digestible format, such as:
- Dashboards
- Heat maps
- Word clouds
- Trend analyses
Practical Applications in Sales and Marketing Operations
Customer Sentiment Analysis can be leveraged in various ways to improve sales and marketing strategies:
Application | Benefit |
---|---|
Product Development | Identify features customers love or dislike |
Campaign Optimization | Gauge reactions to marketing messages |
Competitive Analysis | Compare sentiment towards your brand vs. competitors |
Customer Service Improvement | Identify common issues and improve response strategies |
Lead Scoring | Incorporate sentiment data to refine lead qualification |
Challenges and Considerations
While powerful, Customer Sentiment Analysis comes with its own set of challenges:
- Context Interpretation: Sarcasm and cultural nuances can be difficult for AI to detect
- Data Quality: Ensuring a representative sample of customer feedback
- Privacy Concerns: Balancing data collection with customer privacy rights
- Continuous Learning: Regularly updating models to adapt to changing language patterns
Implementing Customer Sentiment Analysis
To effectively implement sentiment analysis in your operations:
- Define clear objectives for what you want to learn from the analysis
- Choose the right tools or platforms that align with your business needs
- Ensure you have a diverse and representative data set
- Combine automated analysis with human insight for best results
- Act on the insights gained to improve customer experience
By leveraging Customer Sentiment Analysis, businesses can gain a deeper understanding of their customers' needs and preferences, leading to more informed decision-making and improved customer satisfaction. 🚀
As you consider implementing Customer Sentiment Analysis in your organization, ask yourself:
- What sources of customer feedback are we currently overlooking?
- How can we integrate sentiment data into our existing CRM and marketing automation tools?
- What specific customer experience metrics could we improve with sentiment insights?
- How might we use sentiment analysis to personalize our sales and marketing approaches?